BEing a data scientist
Authors: Thom Ives, Manpreet Budhraja, Abdul Najeeb
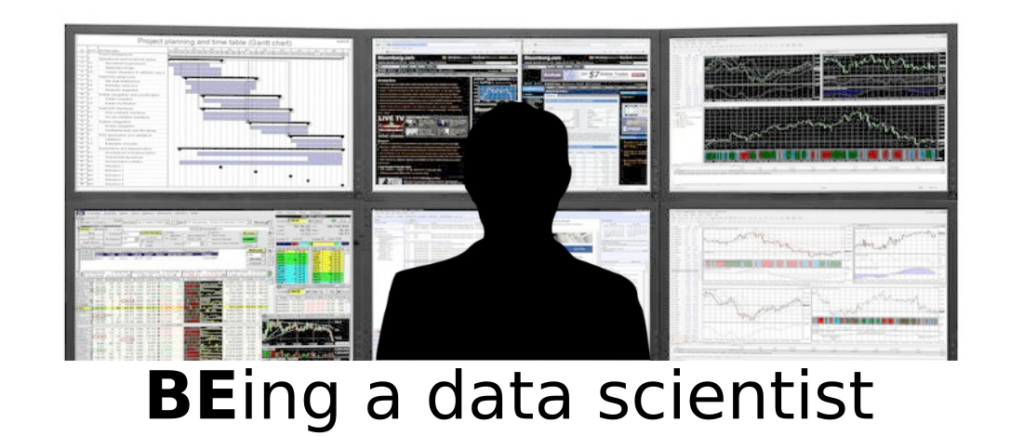
Overview
This may be the most needed post we ever publish in our mentoring category. We’ve read many strong comments regarding landing data science roles. We feel your pain. It’s a tough ordeal to go through on both sides: interviewers and candidates. Candidates can sometimes feel like their part of some modern slave auction. Others can feel like prisoners in the Stanford Prison Guard experiment. Interview teams are overwhelmed by the number of candidates to consider for a single role. Some are empathetic. Some are harsh. One thing is certain. Not getting any response is most likely due to recruiter overload than recruiters not caring. The situation is not likely to improve soon.
We’ve thought long and carefully about how to write this post. We feel strong empathy for all as we considered the many comments that we’ve read. In most of those comments, we felt like a vital spirit was missing. Then we noticed a response theme, a spirit, similar to our own.
We feel that we should encourage and nurture this spirit. We see it as the most essential spirit that prospective data scientists need to adopt. The philosophy of that spirit is explained in the next section.
We will then share a fictional example to illustrate this spirit using Ta’s family. In case you don’t know Ta, Ta is a mythical, yet realistic, example of an early career data scientist. For the remainder of this post, we will share real-life stories of those that live this spirit. We sincerely hope that this post inspires, motivates, and helps you.
Overriding Philosophy
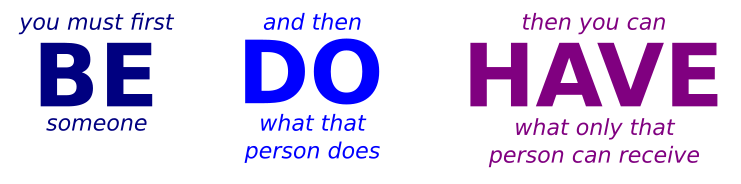
Many people make the mistake of thinking backward about their career – even their lives.
This is the way backward thinking goes.
I must first HAVE things to DO things to BE a thing.
It has been proven that this does not work.
Here is the correct order of thinking
You must first BE to DO to HAVE
What does this mean in our context of data science? BE a data scientist. DO data science that serves needs wherever you are. Then you will HAVE recognition as a data scientist from others.
Let’s go over an example. You’ve completed a modicum of data science training. You’re eager to apply it to business. You encounter massive competition in the job market. Ugh! What’s happening? You have to eat! You have to pay rent! Let’s turn this into a good drama.
Ta And Ta’s Siblings
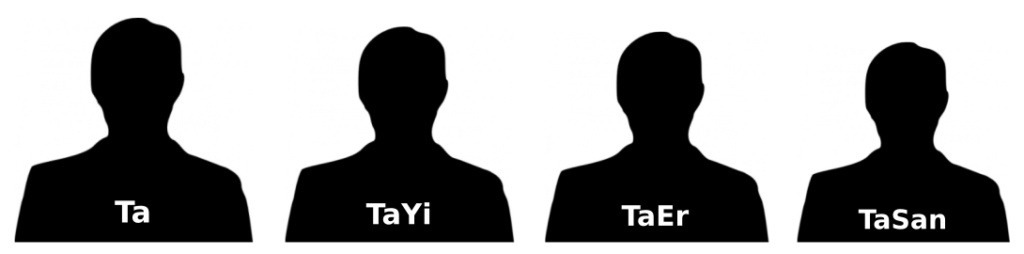
In case you’ve never met Ta before, Ta is a great, early career, data scientist. Ta seeks mentorship from Thom but is now also close to the other members of the Integrated family. In this post, we learn more about Ta’s family. We’ve previously met Ta’s dad and two uncles. In this post, we meet Ta’s three younger siblings. All of them want to be data scientists like Ta.
Ta’s three siblings are close in age. Their names are TaYi, TaEr, and TaSan. You guessed it! Mandarin for Ta-One, Ta-Two, and Ta-Three. Ta is doing great in Ta’s career. Ta’s siblings decide to seek advice from their oldest sibling Ta.
It’s not that they don’t like to get advice from their mom and dad and aunts and uncles. It’s just that they relate to Ta’s use of Batman explicatives better than theirs. That’s not fair, but we must progress with this story.
While close in age, TaYi is the oldest, TaEr is the second-oldest, and TaSan is the youngest. All of them have completed their training in data science. TaYi has landed a role with the title of junior data scientist. TaEr was struggling to find a data science role, so he took a role as a data engineer. TaSan took a job as a waiter at TaSan’s favorite restaurant. Which one has a real data scientist spirit? Let’s find out!
TaYi is explaining to all of them that TaYi is doing tons of data wrangling. TaYi is doing data cleansing, data formatting, feature analyses to do feature reduction, etc. TaYi is doing tons of web searches to learn how to deal with these challenges. TaYi never learned any of these things in TaYi’s coursework and studies. He’s able to figure out how to do these things, because he took Ta’s advice. He became VERY good at python and pandas. TaYi only thought TaYi knew SQL. Thank God for the query designer in SQL Server Management Studio. But THANK GOD even MORE for David Langer’s EXCELLENT SQL courses on YouTube! TaYi is also grateful that the database owners understand TaYi’s need for their help. He asks Ta, when will I get to do data science work? Ta smiles and suggests they listen to their other two siblings first.
What they didn’t realize until just now is that Thom has been listening over a virtual meeting. He interjects with a hello, and they all startle and then smile and say, “Hi, Uncle Thom!” Thom asks, “OK if I continue to listen in?” “Of course,” they all assure him. Ta can tell by Thom’s look that he is trying very hard to suppress laughter. Ta thinks, “Holy déjà vu Batman! I know that look!” He catches himself and says, “Let’s hear about you, TaEr.”
TaEr is very disappointed. TaEr had to take a data engineering job. It’s great pay and engaging work, and TaEr likes TaEr’s teammates. But TaEr feels like it’s a failure. TaEr wants to be a data scientist! TaEr desperately wants advice from Ta and Thom. However, Ta and Thom both want to hear from TaSan before they respond.
So TaSan begins. It’s obvious that TaSan is ecstatic to share about TaSan’s new job. TaSan explains that TaSan landed TaSan’s dream job and starts Monday next week. The pay is low, but the ceiling is super high. It’s a progressive business that has no data scientists. TaSan explains, “I have the opportunity to build the data science program from scratch!” “I will act as their artisan data scientist”, TaSan concludes.
They all look at TaSan in astonishment. Ta and Thom just smile. They already know. TaYi and TaEr ask, “How did you get that job? What’s your title?” TaSan looks at them, a bit confused.
“Title? What’s it matter what my title is. I know I’m a data scientist.” Then TaSan proudly adds, “I’ll be a waitperson at my favorite Asian restaurant.” “It’s a national chain too, so I’ll have plenty to do.”
TaYi and TaEr are speechless. They want to be nice, but what do they say? They are very embarrassed for their youngest sibling. Then they hear Ta say over the silence of their stunned faces, “That’s awesome!” They also notice Uncle Thom beaming with pride! Is he about to cry? “Holy Cry Baby Batman! That guy does get touched easily!” TaYi and TaEr think. But they are confused as to why Ta and Thom are excited for TaSan. “TaSan, I think you get it! But please explain your thinking to us. I want to make sure.”
TaSan explains TaSan’s pay and budget and the excellent free food motive. TaSan further explains that TaSan wanted a position with a growing company that TaSan loves. TaSan will take careful notes on all of TaSan’s observations, and TaSan will first work to ensure that TaSan’s work is respected and appreciated. At this point, TaSan will work to learn the business pressures better from TaSan’s manager. TaSan will also seek to learn from TaSan’s restaurant’s owner and the bookkeeper. TaSan will collect all the data that TaSan can from day one. When necessary, TaSan will create a database. TaSan will visualize that data in many ways and look for stories and helpful analytics.
Ta tells TaSan, “You get it, TaSan. You ARE a data scientist. You will, therefore, DO data science. Once you’ve done good data science for your company, you will HAVE their regard as their data scientist.” “Artisan data scientist!” TaSan corrects. Ta replies, “I stand corrected.” “But you’re sitting now”, replies TaSan. They both just smile.
Thom interjects, “TaEr, I am jealous of you! What an extraordinarily great first role. That role will help you to become a phenomenally good data scientist.” “Really?” TaEr asks. “Absolutely”, answers Thom. “Many of the best data scientists started in Data Engineering!” TaEr replies, “Wow, now that I think about it, that makes a lot of sense. I feel better already. I am thrilled that I took this data engineering role.” Thom and Ta both confirm that both TaEr’s new role and attitude toward it are great news.
TaYi then says, “Wow! I thought I was the luckiest one here tonight. Now I feel like I got the short straw!” “Why?” they all ask. “It feels like I am off to the worst start now.” Thom and Ta quickly object. They assure TaYi that he is already actually doing data science. But he’s confused why he’s not doing any modeling yet. Ta and Thom take turns explaining real data science work to TaYi. Approximately 80% of data scientists’ time is spent on data wrangling – maybe more. Data is not collected in a format ready for modeling. It’s also vital to deliver many helpful visualizations to fellow data scientists and business partners on the path toward predictive modeling.
They talk for at least an hour on the true spirit of BEing effective data scientists. They wrap up with some fun stories and some more explicatives. Wow, uncle Thom is even skilled with Bill & Ted explicatives! Oh, the original movies came out when he was young. One thing is for sure, TaYi and TaEr are happy with their career beginnings now. They even give several encouraging words to TaSan for having a great data science spirit.
Real-Life Stories
The Story Of Andrew Jones
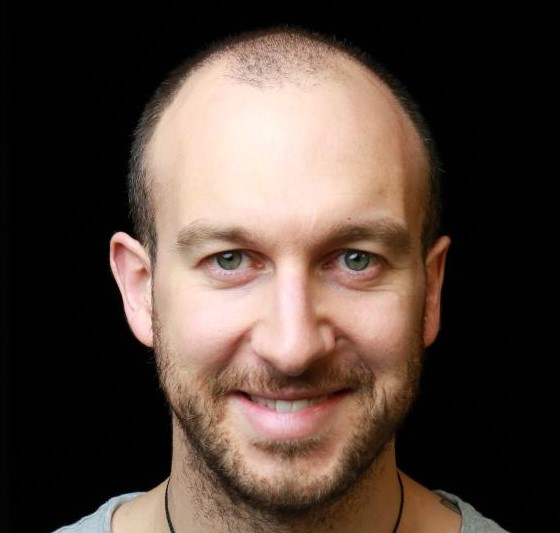
Data Science Infinity
Andrew started in a non-analytics career. He played cricket with an analytics manager and learned SAS to help with club tasks. This exposed him to machine learning – he loved it! He moved from SAS to R to Python. He then worked for Amazon. He even worked on patented ML features for PlayStation 5. Summary? He became an experienced data scientist and is now giving back.
How? The market is flooded with aspiring data scientists. The result? A dream role? Many struggle to land any role at all!
Andrew now teaches people in his program the skills & concepts hiring managers want. He has extensive experience interviewing data scientists for roles at top companies. He’s talked to many leaders, hiring managers, and recruiters. These people interview & screen hundreds of candidates at major companies.
Does Andrew agree with the BE, DO, HAVE spirit toward data science? Absolutely!
The Story Of Gilbert Eijkelenboom
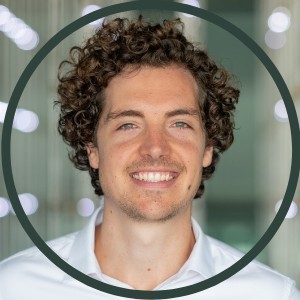
Author: “People Skills for Analytical Thinkers”
Founder: MindSpeaking
Gilbert never considered himself a data scientist. He worked mainly in consulting on analytics projects. He was frequently dealing with questions like: how can we get more value out of data,
how can we use customer data to create predictions about their behavior, etc.?
Gilbert was in many analyst roles and completed many SQL and R courses. He hadn’t been a data scientist in the way that most people think about data scientists. He became fascinated with data science, because he liked the way that data scientists communicate with business team mates. Understanding both sides helped him get a great start in data science. As a result, Gilbert now helps data scientists communicate more effectively. Gilbert has written a book on this subject.
Upon explaining the purpose of this post to Gilbert, he felt it would be very beneficial. He wants people to find jobs that will help them grow their data science skills. He understands that not all first roles can be data science roles. Again, this is his story too. However, many roles can help one grow their data science skills. No one role will prevent you from working on data science type efforts that help business. Just keep up with your primary duties. Gilbert was happy to contribute to this effort.
As we talked, he agreed that it was not essential to get a data science job immediately. If you need income, take the best job that you can get. In other words, don’t starve. Once your regular duties are going well, apply data science principles to improve that business. He agreed that BEing a data scientist was not dependent on you having that title. Just BE a data scientist wherever you are. Have this spirit and DO data science work to benefit your business at every opportunity. You will be far more likely to HAVE a significant data scientist role this way. Your current company may even change your title and pay!
Gilbert wholeheartedly believes in this level of entrepreneurship inside one’s company. He explained that many people say, “I don’t get the chances.” The bigger question is, “Are you creating the chances?” We both agreed people shouldn’t stress over landing a data science role immediately. However, data scientists should BE data scientists wherever they are. They should DO data science work as much as possible. Such data scientists will HAVE great data science titles eventually. Gilbert believes this proactivity is vital for people with data science ambitions. Don’t stress over titles. One can BE a data scientist whether a company gives them that title or not. Forget titles. Get the best job that you can. BE a data scientist.
Hold onto the big picture. Contribute to the goals of your business with data science. This is a sound long-term strategy for one’s career, one’s company, one’s industry.
The Story of Abdul Najeeb
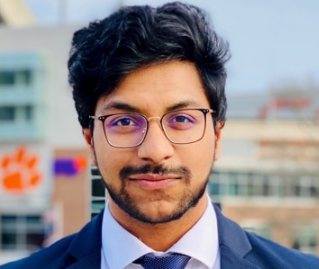
Abdul comes from a background that you wouldn’t consider typical of a data scientist. When he was 20 years old, in 2016, the internet was growing at a steady rate. It was projected to grow exponentially through the third decade of this century.
Moreover, he realized internet growth was being driven by two things: 1) increased usage from people; 2) increased usage of devices needed for services. The growth rate of such devices was exponential. He thought, “Who is taking care of all of this data?” “Who is studying it and seeking to make sense of it and gain use from it?
Was there any roles that did this? A quick google search revealed “data science” was the role. This data was being generated across the globe. Data scientists were at the crux of solving the issues of making sense of this data.
He was pursuing a bachelors of science in mechanical engineering. He considered how well he could help make sense of data as a mechanical engineer? So during his third year of mechanical engineering in 2016, he transitioned to the beautiful field of limitless possibilities data.
The transition was challenging. He had to work extra hard to BEcome as capable as his computer science colleagues. They even started their careers as graduates. He feels fortunate that he was able to join a startup as a junior data scientist. He has learned a lot through this transition. He loves to share this transition experience with the world. He hopes to help people avoid the dry phases of life that he went through in his transition.
The Story of Thom Ives
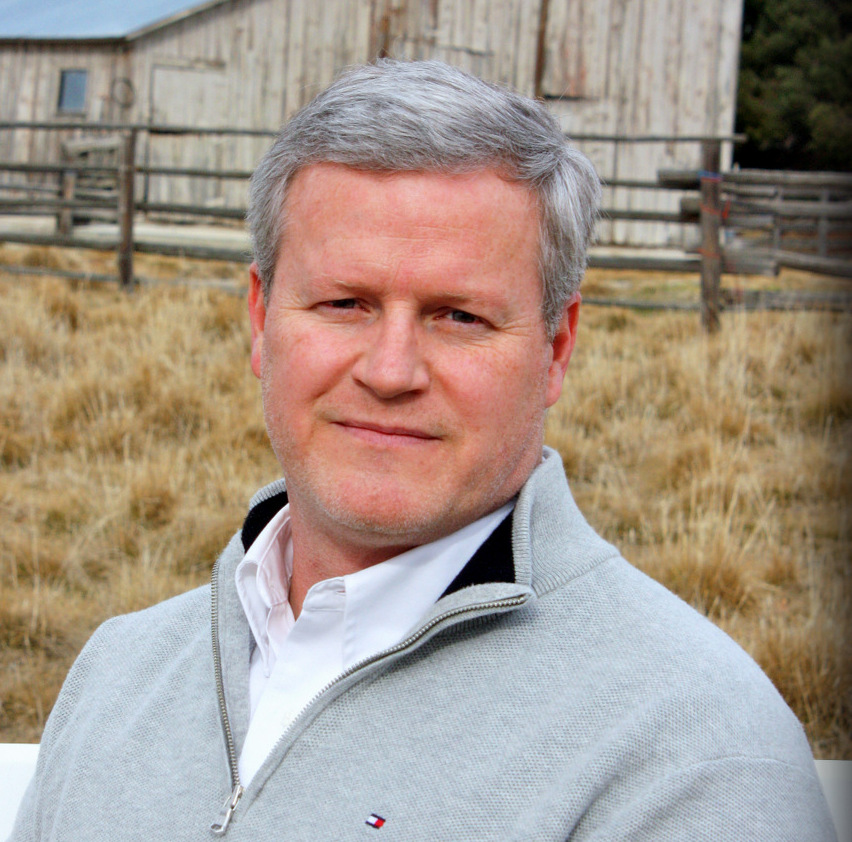
Thom said that this was embarrassing for him to share. He was breaking standard social media protocol. Instead of sharing high points from his career, he chose to confess an ugly chapter from it. But he felt that he could help the community more by highlighting some of his horrible mistakes.
There is an upside to these horrible mistakes. He recovered from them. And he is confident that he will never repeat those horrible mistakes again. If his embarrassing confession helps the community, he’s happy to share it. Early indicators showed him that this confession could help many people. It’s helped many already.
There was a point in his career where he was very angry with one of his past companies. There were times that he contributed greatly there. But his anger and frustration grew to a point where he literally “checked out”. He was only there for a paycheck. He literally stopped caring about doing anything other than collecting a paycheck.
He said that this was not only wrong of him, but it was also blind and ignorant. It caught up with him, and he was in danger of losing his job. It took him time to see the ugly reality of what he had BEcome. He said that he had acted like an entitled brat. He had failed to see that others’ wrong actions could never justify his own bad actions. He robbed myself of valuable self-development time. He robbed his team of receiving his very best work.
Most importantly, he lost a great opportunity. If he could have learned to contribute well in that environment, he would have prepared himself to perform well in a better environment, and
he would have learned skills to help him better serve in another hard environment.
Using this hindsight, he can now see a MUCH better forward path. Always BE great in roles. Always DO great work. Then he can HAVE more chances to make a real difference no matter where he serves.
What’s the happy outcome of that hard and embarrassing chapter of his life? He now works like he tries to code. In code, He seeks to employ clever and elegant practices to benefit his teammates. In his work, he seeks to employ creative and elegant work practices to improve his work habits. In his code, he works hard to wisely deal with coding problems and refactor them to be better. In his work, he is eager to identify his current greatest weaknesses and correct them.
He said that due to the lost self development time during this chapter, he may never make any envelope-pushing contributions to our beautiful sciences. Such honors belong to the likes of Hinton, LeCun, Sutton, and MANY more. However, he will try! He may fall short of his goals to serve the awesome Prospector business at UL, but, he will never stop trying, AND he will seek to make improvements in small helpful steps along the way. He may not bless our great community to the level that he hopes to, but he will try!
The Story of Susan Walsh
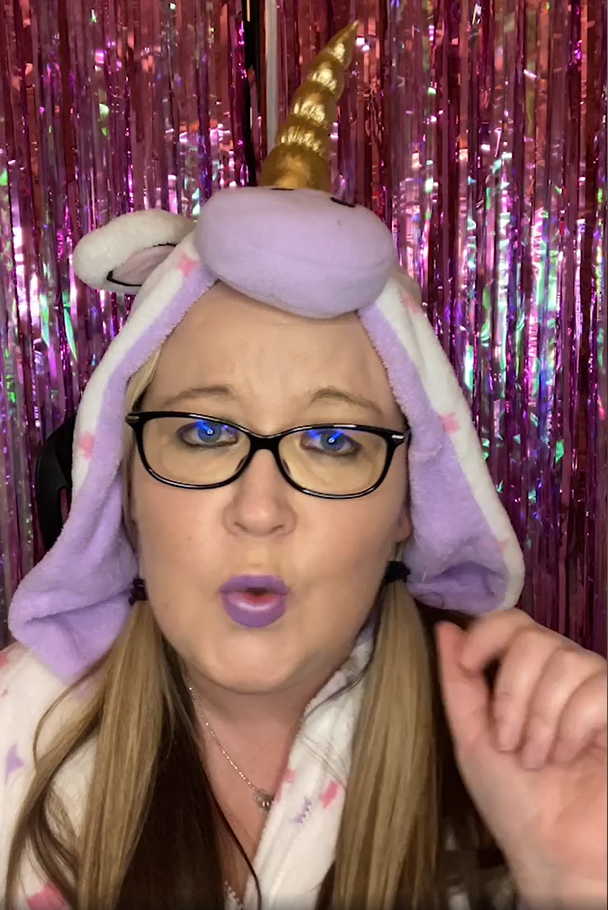
The Classification Guru
40-year-old Susan Walsh, The Classification Guru, has had a colorful career. In three years, she grew her business from a pipe dream. It is now an internationally competitive company. And Susan, an expert in her field, regularly speaks about cleaning and maintaining data. But how did she build her business? It took hard work, determination, and a little bit of luck! We contend that luck happens by being ready when opportunity knocks. Susan is ALWAYS ready!
Susan graduated with a degree in commerce. She didn’t know what she wanted to do when she finished. In her first job, she traveled all over Scotland as a paint merchandiser. But her dream was to live and work in London. Her company had a vacancy down south, which brought her to Guildford. It didn’t last long and wasn’t for her anyway. Then she tried recruitment … that was traumatic!
She tried print sales, telesales and also worked weekends at a nightclub. Then she got a job with Colgate selling toothpaste to dentists. Susan then pushed for a promotion and was sought by several departments. She took a role as a national account executive. However, she was very unhappy in that role and decided to open a shop!
The shop focused on the shift from formal to smart/casual apparel and accessories. Since Guilford didn’t have anything like that, she tried it there. She developed a proper business plan and launched. The shop didn’t profit. Why? She was struck by brand snobbery. She wasn’t Gucci or Calvin Klein. It was tough to adjust to this. By the time she made a positive turn, she was broke. She had to shut the shop, and she was so broke, she couldn’t even afford to go bankrupt.
She had to do something, so she started doing data classification work through Gumtree. Although she’d never done data classification, she gave it a try and took to it very quickly! She was fast and accurate at it. It wasn’t full-time, so she took another job at Wonderbra. She was even on QVC – her 15 minutes of fame.
Before long, she had a full-time role classifying data. As the business grew, she recruited a team and trained them to classify data. She fell in love with data classification.
After five years, she wanted something more. She started her own business as “The Classification Guru!” Susan saw ways to help businesses make their current data more valuable. She consistently classified data twice as fast as anyone else, so the Guru part was natural.
It was scary at first trying to land contracts, but she went all out on marketing. Her advertising videos on LinkedIn helped her a lot. They are fun and popular. What you see of Susan online is what you see when face to face with her. Susan is real and authentic all the time. She even takes time to help new people break into doing what she does.
Susan has crafted great messages about her work, too, that have become quite catchy. Data should be Consistent, Organized, Accurate, Trustworthy = COAT.
Susan’s business is now growing at a steady pace. She’s exploring ways to expand. And even her advertising is popular.
Susan encourages people to experiment as she did. All those experiences helped her grow. And her new experiences are helping her grow even more now. She’s producing increasingly more podcasts, webinars, and blogs. She’s laying the groundwork for growing her business. She’s planning to hire additional people soon to help with her ever increasing workload.
The Story of Manpreet Budhraja
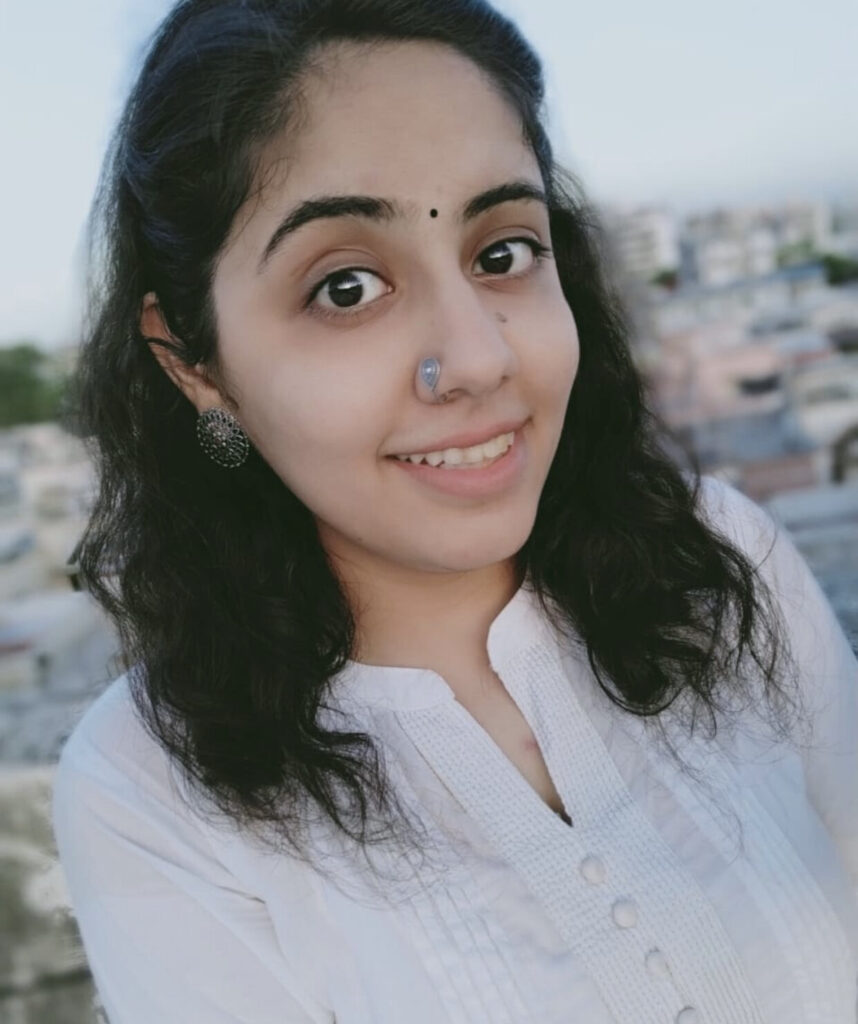
Manpreet’s journey did not go according to her original plan. She started out in mathematics. Now she works as a Data Engineer, and she will always BE a data scientist. Her story is much like TaEr’s.
In college, she wanted to do more than math. So she developed android applications in a college club. That led her to web development, and that led to exploring fields within data science.
She was excited that her mathematical studies prepared her very well for data science. To develop further she did various unpaid internships and attended summer school. And she grew a vision to be part of and to help build a data science family. Her career has been further enhanced by her hunger to learn and her willingness to take risks.
While nearing completion of her M.Sc. in Applied Mathematics, she received a job offer. However, she wanted more growth than that job could provide. So she started networking with people on Linkedin and seeking ways to grow. Besides sharing her own views, she listened to others’ views. And she took every opportunity to learn from others.
After many struggles, she took a job as a data engineer. She was quite hesitant to take that job at first. Now she is very glad that she’s beginning her career in data engineering work. She knows that she will always BE a data scientist. She also knows that good data engineering work is important to her growth. Doing good data engineering will help her become a better data scientist. And she is looking for ways to enhance her data engineering work with data science arts.
She now encourages others to BE great data science community members. Therefore, she seeks to help others through her posts, blogs, and videos. She now encourages everyone to:
- never lose hope,
- work hard for their vision,
- network and communicate,
- practice the theory of “Learn-Unlearn-Relearn” to continually grow.
A Compliment to Michael Green
On LinkedIn, Michael asks why data science candidates expect to get a job based on theoretical knowledge? Should they show up for a job interview and:
- Brag about how they can solve a double integral without consulting the internet?
- Sit down at a whiteboard and write down a memorized procedure for solving double integrals?
He advises that they should instead:
- Convince the hiring manager that they understand the problems that their team faces.
- Demonstrate that they can help solve these problems by applying the right tools in the right way.
- If the problem really does require a double integral, they should explain how they used them to solve similar problems.
He empathizes with those who are not getting traction in their job search. But he’s not surprised. Most materials are only teaching data science theory. Candidates have a false hope that this is sufficient to get their first job. Coding SVMs from scratch doesn’t cut it. They must convince hiring managers that they are capable of solving relevant problems.
A Compliment to Jonathan Tesser
On LinkedIn, Jonathan explains that he wants to know how candidates can alleviate the business’s pains. Jobs are available, because there’s not enough people to finish the current workload. They’re available, because there’s a need to do things, and no one to do them. The person that best demonstrates that they can reduce the business pain gets the job. So concentrate on finding out their pain and showing how you can reduce it. The candidate that is most convincing in being able to reduce the pains gets the role.
Summary
BE a data scientist by thinking like a data scientist – always. DO what data scientists DO. They look for ways to solve problems and to reduce pain using data and data science. As you improve your skills at this, you will HAVE the recognition of BEing a data scientist. This earned reputation does not depend on your job title. Such accomplishments can go on resumes. Such resumes can land roles with data science titles.
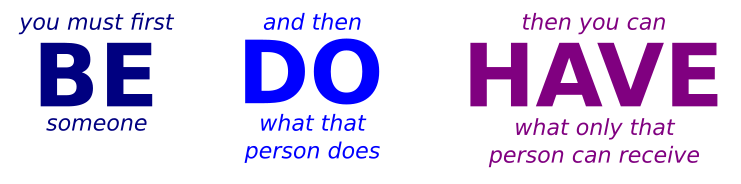